The market of AI in the healthcare sector is booming. It is expected to reach almost 28 billion U.S dollars by 2025. Today, AI for diagnostic predictions can be used as a system for making medical and management decisions in the health industry. How exactly does AI shape the healthcare sector and what are the frontline solutions?
AI for Diagnostic Predictions
Predictive analytics is a technology that relies on large amounts of data to predict certain outcomes. It uses many methods from statistics, and data mining, and takes into account both current and historical patients` data making predictions based on it.
In healthcare, such a system analyzes medical records, extracts important clinical information with the help of natural language processing (NLP) technologies, and then predicts the occurrence of serious health problems in the near future.
The system allows analyzing a variety of patient health data using big data.
The range of tasks can vary greatly and every solution is developed based on the set goals. It can include anything from the decision support features to analytics that helps scientists and doctors to make preventive and health management decisions.
There is an avalanche of applications for it: it helps conduct real-world clinical practice research, offers custom development of algorithms and machine learning models tailored for a particular task, and other research work. It is highly adopted by research organizations, contract research firms, and pharmaceutical companies.
Such a platform helps doctors better understand possible negative events with the patient’s health in the future and detect diseases at an early stage. This makes it possible to provide preventive medical care, as well as effectively organize the prevention of chronic diseases and reduce mortality.
Using the system as a supportive tool makes it possible to identify risk factors and a high probability of developing diseases.
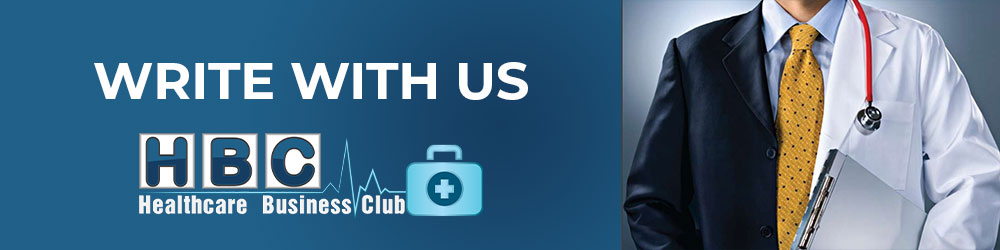
AI in Medical Imaging
According to Global Outlook & Forecast 2022-2027, AI in medical imaging is expected to surpass 10 billion U.S dollars by 2027.
The technology primarily uses deep learning to collect useful information from X-rays and use it to make clinical decisions. Deep learning refers to the process by which a computer takes data and then, based on the broad knowledge gained from analyzing other data, interprets that information.
Special methods for processing large data sets that are generated during the analysis of medical images allow physicians to develop a personalized treatment plan for a particular patient by accessing a cloud-based solution.
Here are some of the benefits AI and ML provide for medical image analysis:
- With improved AI and ML-based medical image analysis, medical experts can improve the productivity of their clinical process and work productivity.
- These technologies reduce the risk of incorrect analysis and delays, increasing the reporting, interpretation, and reading of results.
- Medical teams equipped with AI and ML-based medical image analysis systems can make informed and quick decisions, easily diagnose diseases and optimize workflow. This ultimately improves patient satisfaction and experience
- AI and ML will enable researchers to analyze data and find better treatments for new diseases. In addition, deep machine learning technologies can generate new data from training data, automating the learning process.
The best thing about this technology is its growing precision. Computational capabilities and advances in ML algorithms enhance the capabilities of image analysis.
For example today AI models show an outstanding precision for detecting COVID pneumonia with an average accuracy of 95%.
Even today, artificial intelligence allows professionals and experts to automate and generate medical analysis without wasting time. In the near future technology will surely become more precise and improve the quality of medical imaging.
Cancer Prediction
The Cancer Genome Atlas project has been implemented by the U.S. National Institutes of Health since 2005 to systematize data on genetic mutations that lead to cancer. It involves the study of mutations in the genes of 12 tumor types and analysis of other molecular characteristics.
Scientists use AI for diagnostic predictions and to borrow treatments for one type of cancer from the arsenal of therapies for another one.
By comparing cancer characteristics with clinical manifestations as well as with external factors AI makes it possible to:
- create the most complete biomedical models of normal and pathological processes, and identify associations;
- construct diagnostic predictive tools;
- develop new treatments and improve the effectiveness of treatment strategies and tactics, e.g., through targeted therapies;
- reduce high costs of health care, for example, by comparing the clinical effectiveness of different originator and generic drugs;
- help the medical professional make decisions in the face of enormous amounts of information and a multiplicity of conceptual explanatory models;
- improve medical classifications, for example, to take into account the varying degree of dependence of pathology on certain factors, the multifactorial nature of normal and pathological processes, and their dynamism.
A case in point could be the FocalNet system, a convolutional neural network, which identifies prostate cancer. The accuracy of this AI-based system is 79% compared to humans’ 80%. That equips radiologist with a promising tool that helps them identify potential tumor before it develops.
Read Also
- Optimizing CT Protocols: The Hidden Key to Efficiency and Cost Savings in RadiologyIntroduction: Why CT Protocol Optimization Matters Computed Tomography (CT) is a cornerstone of modern diagnostic imaging, providing critical information across nearly every medical specialty. However, maximizing the value of CT — both clinically and financially — requires more than just advanced hardware. The real secret lies in the optimization of CT protocols. When CT protocols… Read more: Optimizing CT Protocols: The Hidden Key to Efficiency and Cost Savings in Radiology
- The Role of Carbide Burs in Modern Dental ProceduresAs a result of this procedures need to be well coordinated and to this end, precision tools are used by dental practitioners. Among the most essential tools in a dentist’s arsenal are carbide burs, which have revolutionized various aspects of dental work. Today’s dentistry cannot work without these tools as they are both strong, sharp,… Read more: The Role of Carbide Burs in Modern Dental Procedures
- Detection of Diabetic Retinopathy: The AI AdvantageDiabetic retinopathy (DR) is a leading cause of blindness among working-age adults, affecting millions worldwide. The prevalence of DR is alarmingly high, affecting an estimated 34.6 million people globally. In the United States alone, it is estimated that 7.7 million adults have some form of diabetic retinopathy. How Does Diabetes Affect the Eye? Most of… Read more: Detection of Diabetic Retinopathy: The AI Advantage
- Advances in Digital Health: Transforming Modern HealthcareThe healthcare landscape is undergoing a seismic shift with the advent of digital health technologies. These innovations are not just modern conveniences; they are transformative tools that bridge gaps in accessibility, improve patient outcomes, and empower individuals to take charge of their health. From wearable devices to telehealth and artificial intelligence, digital health is creating… Read more: Advances in Digital Health: Transforming Modern Healthcare
- The Latest Advancements in Urology ProceduresUrology, the branch of medicine concerning the urinary system and male reproductive organs, has seen remarkable advancements in recent years. Technological innovations have led to more precise, less invasive, and increasingly successful procedures that promise better outcomes for patients. From state-of-the-art robotics to personalized medicine approaches, the field of urology is evolving at a rapid… Read more: The Latest Advancements in Urology Procedures
Drug Discovery
Apart from predictive analytics, research, and medical imaging, AI also helps in drug discovery. In fact, this area shows the most growth and market size. By 2025, the drug discovery market is expected to reach $3 billion.
Basically, the algorithm is based on the same data analysis.
To find the right drug, scientists have to find a molecule that can cope with the disease.
This presupposes finding molecules with the required properties, predicting their properties, and synthesizing them.
The problem lies in the number of molecules that can have medicinal properties. Theoretically, 1033 molecules could have medicinal properties. That’s a huge number. However, the largest databases contain hundreds of millions and even billions of molecules with described properties.
Drug engineering requires a cutting-edge algorithm that can find a needle in a haystack.
One of the solutions is artificial intelligence and virtual screening.
According to the article in Expert Opinion on Drug Discovery journal, machine learning methods are much less likely to make mistakes and work better than humans. In addition, virtual screening helps pharmaceutical companies reduce time, resources, and errors in drug development.
Screening out inappropriate molecules with the help of AI saves a lot of time since it is much easier to screen the remaining molecules.
The virtual screening approach boils down to the fact that we test a huge number of molecules on the AI model, which saves time and reduces errors during drug engineering and testing.
AI Challenges in Healthcare
AI-assisted research and hybrid AI systems tremendously support physician decision-making.
However, to fully uncover the potential of AI in healthcare, more freedom for scientists and healthcare professionals is required on a legislative level. Data privacy also plays a huge role.
Obtaining data in a single standardized format will accelerate the process of creating IT solutions, new drugs, and treatments.
A complete picture of the health of the population will enable the automatic accumulation of more accurate statistical information by region and disease, as well as the calculation of correlations with specific socio-economic conditions and the more efficient allocation of resources.
In Denmark, for example, there is an open disease history for the past seven years with the International Classification of Diseases codes and any researcher can use them.
However, the problem of disparate data is common in all countries.
For example, data on mortality may be considered public in one country and private in another. The degree to which electronic health record data are formalized and structured also varies in many countries.
Developing ways to standardize stored data is another industry trend.
For example, the contact tracking system from Apple and Google allows you to find out anonymously whether the owner of a gadget has had contact with a person sick of coronavirus and how high the chance of infection is.
Once data regulations will be standardized it will be easier to collect and combine large amounts of scientific knowledge and clinical practice for the development of medicine and pharmacy and improve the efficiency of management decisions in healthcare.
Until then, the problem with the accuracy of AI systems and their effectiveness will be up in the air.
However, there are already many AI-based platforms where users can view their data and share it with consulting physicians.
They help healthcare professionals to summarize poorly formalized and contradictory data from different areas of medicine, which simplifies treatment.
In Conclusion: AI in Medicine – Challenges and Opportunities
In addition to the obvious benefits that AI has to offer for healthcare, there are also some downfalls.
The main problem is that there is currently no single technical standard for generating medical histories that would allow for the integration of all of this data.
The second point is the risk of data leaks. As we know, information leaks are quite common today, so a reliable protection system is of foremost importance.
Thirdly, the technology is far from perfect and has more “complementary nature”. AI in medicine and pharmacology greatly simplifies the lives of doctors and patients, but only provided careful implementation of this system to the company.
Artificial intelligence works according to the “black box” principle: if there is an error in the algorithm and the system makes a wrong decision, it will be difficult to figure out the problem.
In addition, new technology is not cheap. Many clinics and hospitals will not be able to implement them due to a limited budget.
Nevertheless, there is no denying that artificial intelligence is a promising technology that is already being implemented in predictive practices and proving its effectiveness. There is no doubt that in the future it will be used in all medical institutions, improving the quality of diagnosis, treatment, and prevention of various diseases.