Overview
The rapid developments of AI in radiology has led some to argue that artificial intelligence may one day replace human radiologists.
Researchers have used deep learning to develop neural networks that can identify abnormal findings in medical images such as fractures in X-ray images and possible cancerous nodules or lesions, and in some cases, this is more reliable than a beginner or moderate-level radiologist’s reading.
The best AI radiology solutions today are almost equivalent to human performance and present very promising and reliable results but they are only used in research settings and are still no widespread in field healthcare.
Deep learning is evolving rapidly, and it is a much better technique than previous methodologies that were used in medical imaging analysis. Perhaps this heralds a future in which AI plays an important role in radiology.
The radiology field will certainly benefit from systems that can read and interpret multiple images quickly, as the number of images has increased significantly and rapidly in the last decade, surpassing the number of radiologists themselves.
Hundreds of medical images may be generated for a single patient in a single scan of CT or MRI regarding his illness or injury.
Because medical imaging services are very expensive, any solution that can reduce human effort, reduce costs, and improve diagnostic accuracy, will benefit patients and clinicians alike.
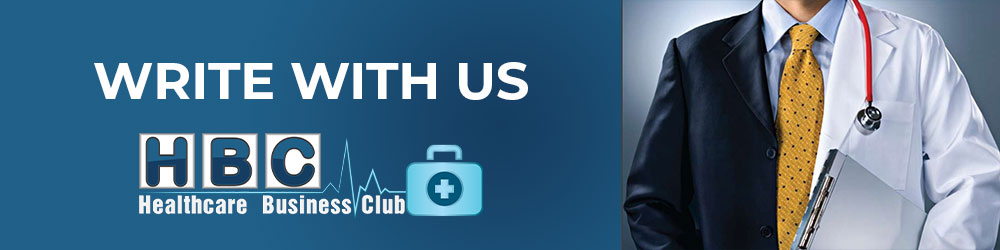
Is AI in Medical Imaging Shaping a Threat for Radiologists?
The new generations of medical students might refuse to specialize in radiology despite the shortage of radiologists in Middle East and Africa, they might think that Radiology career is not promising because of AI and will disappear someday as this technology will read, interpret, and report medical images.
However, we are confident that the vast majority of radiologists will continue to occupy their jobs for decades to come, AI is not causing a threat to radiologists but it’s empowering them and making their workflow faster and with higher quality.
Artificial intelligence in medical imaging is not going to replace human radiologists or will never be an alternative for the upcoming decades in the Middle East and African zone.
There are multiple reasons from my own point of view that would inhibit this incidence and AI in radiology will not shape a threat for radiologists.
Economical Related Reasons
This is one of the most important factors when we talk about the adoption of AI in the Middle East and Africa region.
The African continent countries are all classified to be developing countries or underdeveloped, many countries are still struggling with the basic healthcare services like access to care, quality of care, medical equipment, physicians, specialists, new technologies, ….etc.
The list is so long when it comes to mentioning the healthcare settings in most of the African countries, we are still trying to obtain the basics of healthcare equipment and service levels.
In the field of radiology in Africa, there is a big shortage in radiologists within many countries and radiology equipment as well.
Most of the facilities now are thinking of buying basic requirements of radiology equipment including X-ray, Ultrasound, CT, and MRI machines, especially in the challenging and rising burden of healthcare costs because of the Covid-19 pandemic.
The trend is going to develop teleradiology services to compensate the shortage of radiologists and deliver the reporting service to suburban and rural areas.
Adopting AI in radiology would be of a high cost which will be more limited in most of the African region and if used it will be used in a very limited scale.
We might see some other rising economies in the Middle East like UAE, and KSA are early adopters of this technology as they have the infrastructure, equipment, and financial capabilities for implementing this technology. But even when applying this technology, it will not be generalized for the whole country.
These economical factors mentioned, can make us conclude that it’s not easy at all to go without a human radiologist.
Actually, we are trying to compensate the limited supply of human capital in radiology with the communication technology and enable them to provide the service remotely to multiple cities or even countries through teleradiology which is one of the Tele-healthcare services.
Read Also: Artificial Intelligence (AI) in Healthcare Industry, Ultimate Guide
Technology Related Reasons
In this section, we will mention some details about the AI technology itself in medical imaging and if it is really fulfilling all the requirements to go without a radiologist.
Radiologist is Doing More Than Image Analysis & Reporting
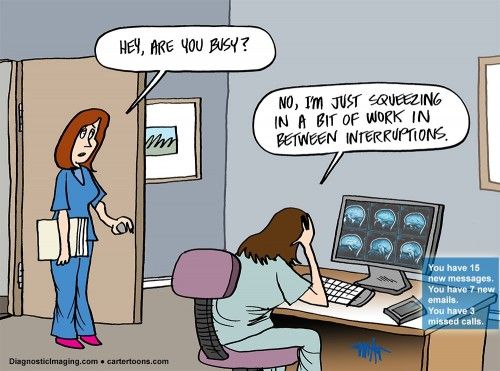
What a radiologist does goes far beyond just reading and interpreting medical images.
Artificial intelligence systems in the field of radiology, like other artificial intelligence systems in other fields or industries, perform specific and limited tasks without the holistic vision of the situation and this is called limited artificial intelligence.
- Advances in Digital Health: Transforming Modern Healthcare
- The Latest Advancements in Urology Procedures
- Navigating the Future of Healthcare: The Role of Technology and Innovation at Saint Luke’s Hospital
- 8 Tips For Aligning Optical Systems In Medical Devices
- The Evolution of Orthodontic Technology: Exploring the Innovations Behind Modern Braces
The current deep learning models have been trained for specific tasks in medical image analyses to detect the potential abnormalities in a CT or MRI exam as an example.
All tasks of AI in medical imaging right now are set to detect a limited number of diseases even without correlation with other studies or patient history which is important to give a better diagnosis and discover the real reasons behind the images’ findings.
Moreover, the task of interpreting medical images does not include just one set of tasks that radiologists perform.
Diagnostic Imaging is a dynamic process where radiologists also exchange advice with other radiology consultants and referring physicians about diagnosis and treatment.
In some cases, like interventional radiology as an image-guided procedure, the technique of the operation is defined according the case of each patient and linking results from the medical images, medical records, patient history, and lab results with the required procedures.
No Integrated & Interoperable AI solutions in Medical Imaging Yet
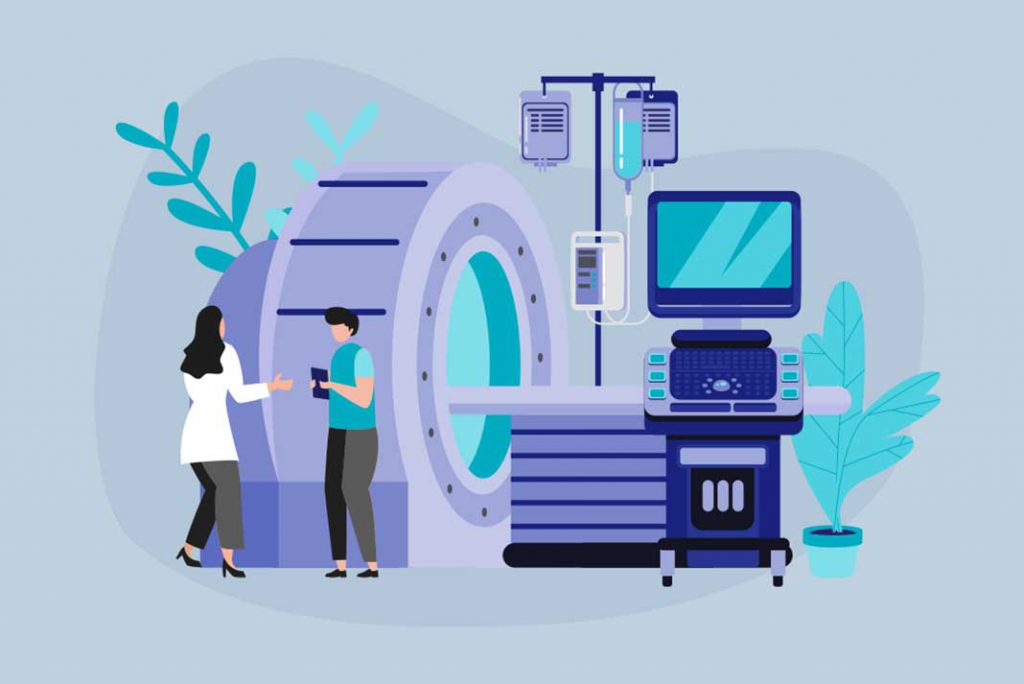
The application of AI-based technology in medical imaging are still far from the application in the clinical settings for the everyday use.
The current imaging technology vendors are still developing deep learning algorithms for specific aspects with different focus on probability of diseases, locations of lesions and nodules and different body parts.
that companies supplying different radiographic techniques and deep learning algorithms focus on different aspects of the use cases they study. Even among the deep learning-based nodule detectors approved by the FDA, there were different focus areas: the likelihood of pathological lesions, the likelihood of cancer, the feature or location of the nodule. These distinctive focus areas make integrating deep learning systems into current clinical practice extremely challenging.
Also, the usage of Ai-based medical imaging systems requires FDA approval, all technology supplier companies must verify the effectiveness of the designed algorithms before taking these systems to the market and this process takes a lot of time.
The production of comprehensive systems that have integrated algorithms which can interpret and correlate medical images from different body parts and other imaging studies would take a very long time as the existing systems are still handling specific modalities, diseases or use cases.
What was mentioned in this point assures that the need for radiologists is a must have asset, the clinical practice cannot go without the existence of human radiologists for many upcoming years.
The Availability of Big Datasets
Deep learning algorithms must be trained on “labeled data” (data subject to classification by people in advance).
In radiology, this means a lot of studies for patients who have had a confirmed diagnosis of cancer, broken bones, or other diseases.
In other image recognition technologies, deep learning has achieved high levels of success, it has been trained with millions of labeled images using the images of multiple objects available on the Internet.
However, there is no repository that collects medical images, whether labeled or not. These are the property of the supplier companies, hospitals and doctors, imaging facilities, and patients.
The process of collecting massive sets of medical images and labeling them to form a big data to train the deep learning algorithms on them will be a very big challenge, and it will take a lot of time.
The Medico-legal Responsibility
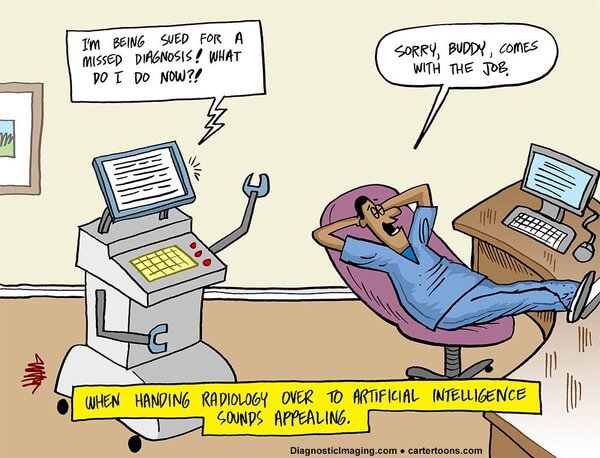
Using AI technology requires changes in systems and regulations to be fully adopted, so there will be a need for changes in medical and health insurance systems and regulations regarding the launch and activation of automated image analysis.
Let’s give an example to outline the situation, who will be responsible in the event of a diagnostic error for a specific case, is it the physician? the hospital, the technology provider, or the data scientist who created the algorithm?
Healthcare & Service Reimbursements
Regarding the payment for services, will those who receive a study report pay for the diagnosis that is made by artificial intelligence as a one-sided process, or is it a two-way process that the artificial intelligence technology performs in partnership with the human radiologist?
What to Conclude
All of these issues need to be resolved, and it is unlikely that any progress will be made in this area as quickly as deep learning research is being conducted in the labs.
It may be necessary for artificial intelligence in medical imaging to outperform the human radiologist and become much better than him, not to be of the same quality, in order to push for the necessary changes in regulatory legislation and to bear the resulting costs.
It should be clear that the next time you have a mammogram using an MRI, it is unlikely that the mammograms will be viewed only by the AI algorithm.
Radiologists will find that their current jobs undergo changes, without being replaced or replaced, just as lawyers, financial planners, accountants, and other professionals see some of the job tasks performed by smart machines.
Given this, they will be required to adopt new skills and working procedures. As stated in a medical futurist blog post, the only radiologists whose jobs may be threatened by AI are those who refuse to work with AI.
Besides, there are intrinsic medical and productivity advantages to be gained from integrating AI with radiology practice.
Productivity improvements may also mean that radiologists can spend more time doing things many of them find it more important like getting second opinion about the diagnosis and treatment strategies.
If the expected improvements in deep learning for analyzing radiographic images occur and are realized and achieved, then the vendors supplying these ai systems, the patients, and payers who pay for the services will be attracted to the radiologists who are able to work effectively with artificial intelligence.